Unlocking the Future: The Importance of Machine Learning Data Annotation
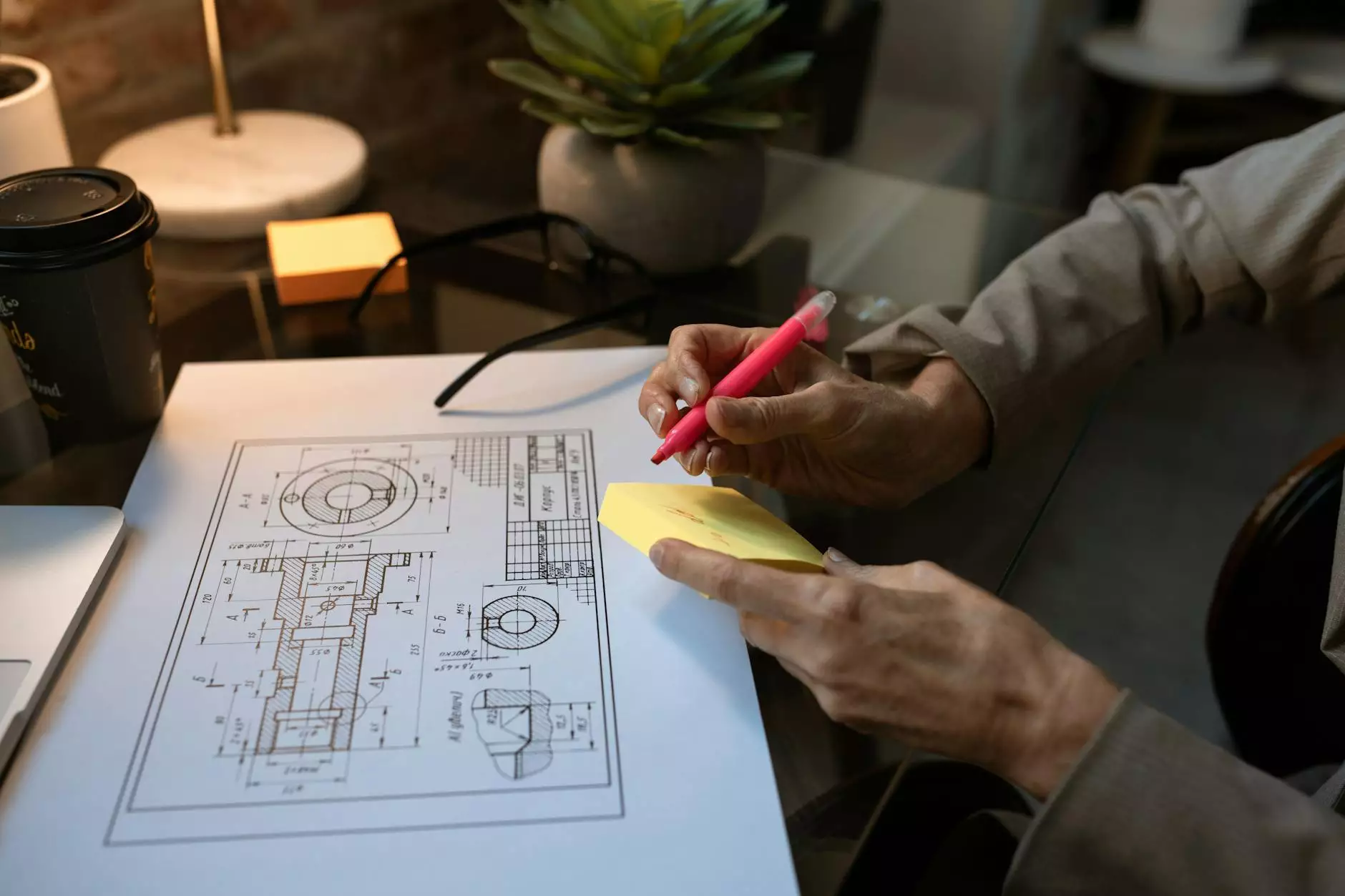
Machine learning data annotation has become a cornerstone in the development of intelligent systems across numerous industries. It serves as the fuel that powers the engines of artificial intelligence (AI) and machine learning (ML). In this article, we will delve into the nuances of data annotation, particularly in the context of home services, keys, and locksmiths, and explore why it is indispensable for the advancement of AI-driven technology.
What is Machine Learning Data Annotation?
At its core, machine learning data annotation involves the process of labeling data to train algorithms to improve their performance in predictive tasks. This process is integral to supervised learning, where models learn from labeled datasets to recognize patterns and make informed decisions based on new, unseen data.
The Significance of Data Annotation in AI
Data annotation enhances the ability of ML models to deliver accurate predictions by providing them with the context they need to understand the data better. For instance, in the realm of home services, AI can be trained to identify different types of locks or detect various locksmithing needs through annotated images and text datasets.
Why is Data Annotation Crucial in the Home Services Sector?
The home services sector has witnessed a surge in the use of AI technologies, making data annotation a vital resource. Here are some key points highlighting its importance:
- Improved Accuracy: Annotated data helps AI systems learn with precision, reducing errors in tasks such as diagnosing security issues or identifying the right locksmith services needed for a home.
- Enhanced Customer Experience: AI solutions powered by well-annotated data can provide tailored recommendations, making the customer experience seamless and satisfying.
- Efficient Service Delivery: Accurate data annotation enables faster response times, allowing locksmiths to pinpoint the issues and offer solutions promptly.
- Better Resource Management: By understanding the traits and nuances of various locks and keys, AI can optimize inventory management for locksmith businesses.
Types of Data Annotation Techniques
Various techniques serve the purpose of machine learning data annotation, each suited to different types of data and tasks. Here are some popular methods:
1. Image Annotation
Involves labeling images with relevant information. In the context of the locksmith industry, this could mean tagging different types of locks, doors, or security systems.
2. Text Annotation
This technique involves marking up text data, essential for training models in natural language processing (NLP). For example, annotating customer reviews or queries to extract sentiments or identify service needs.
3. Audio Annotation
This involves labeling audio files. For locksmith businesses, this can help integrate voice recognition systems that can understand customer requests over the phone.
4. Video Annotation
Labeling different segments of a video can be beneficial for training models to recognize events. Using this in home security could enhance monitoring systems.
Challenges in Data Annotation
Despite its benefits, machine learning data annotation comes with its own set of challenges:
- Scalability: As datasets grow, annotating vast amounts of data becomes an increasing chore, requiring more resources and time.
- Consistency: Maintaining consistent annotation across different annotators is crucial to ensure accuracy, yet difficult to achieve.
- Complexity: For specialized fields like locksmithing, annotated datasets must be handled by experts who understand the nuances of the industry.
- Cost: High-quality annotation services can be expensive, posing a financial challenge for smaller businesses.
Best Practices for Effective Data Annotation
To overcome these challenges and ensure high quality in machine learning data annotation, businesses should consider implementing the following best practices:
1. Define Clear Guidelines
Creating a comprehensive set of guidelines for annotators is essential. This ensures that all data is labeled consistently and accurately, minimizing misinterpretations.
2. Use Annotation Tools
Leverage advanced annotation platforms that provide efficient and user-friendly interfaces, helping to simplify the data labeling process.
3. Continuous Quality Control
Regularly review and audit the annotated data to maintain high standards and rectify any inconsistencies, ensuring the data remains reliable.
4. Involve Domain Experts
Engaging experts in the field of locksmithing for data annotation will yield better results and insights that might be missed by non-specialists.
The Future of Machine Learning Data Annotation
As AI technologies rapidly evolve, so too does the field of data annotation. The advent of new tools and methodologies, such as semi-automated and automated annotation platforms, promises to streamline the process.
Adapting to Change
In the locksmith industry and other home services, firms will need to stay current with these advancements. As businesses integrate more sophisticated AI tools, they must invest in continuous learning and adaptation to maintain a competitive edge.
Conclusion: The Key to Unlocking AI Potential
In conclusion, the significance of machine learning data annotation cannot be overstated. It acts as the foundation for training accurate and efficient AI models that not only enhance service delivery in the locksmith and home services industry but also improve customer satisfaction. As technology continues to advance, businesses that prioritize data annotation will be at the forefront of innovation, driving the future of AI applications in their fields.
By embracing best practices, overcoming challenges, and staying ahead of trends, locksmiths and home service providers can harness the full potential of machine learning, ensuring their services remain relevant in an increasingly data-driven world.